February AI Prompt of the Month: How Many Man Hours Will I Need This Year?
I would bet that you often find yourself in a situation where you wish that you could just run a “quick report” of some business metrics. Assuming you have access to the data, usually it doesn’t take long to realize that reports can be pretty complicated to create. The data analysts (if you have any) probably rolled their eyes when you said the word “quick.” Luckily, AI can do hard things well, and fast! This prompt is just an example of turning a simple ServiceTrade export into a quick report, with a visualization, faster than even an Excel expert could combine a pivot table and a chart together (I know, because I took the data in this example and ran it through Excel using a pivot table and a chart, just to be *sure* the AI wasn’t lying to me).
The Prompt
Given the attached spreadsheet of recurring services that my company provides to my customers, can you create a forecasting visualization of man hours required to complete the services for the next 12 months? Base the date on the “due_next” field and the man hour estimate on the “estimated_duration” field in the data.
The Output
The spreadsheet contains a wide range of columns (140 in total), including the fields id, ref_number, company, company_street, company_city, company_state, company_postalcode, store_number, location_name, and many more, with some fields relevant to your request such as estimated_duration. However, the due_next field you mentioned for basing the forecast on does not appear in the first few columns displayed. I’ll need to locate this field and the estimated_duration field specifically to proceed with creating the forecasting visualization.
Let’s identify and extract the necessary data for the due_next and estimated_duration fields from the dataset.
[AI stops and thinks for a bit here…]
The due_next and estimated_duration fields are indeed present in the dataset. Now, I’ll proceed to filter and prepare the data for the forecasting visualization. This will involve:
- Extracting only the relevant fields: due_next and estimated_duration.
- Ensuring that the due_next field is in a proper date format and that estimated_duration is in a numeric format.
- Aggregating the data by month to calculate the total estimated man hours required for each month in the next 12 months.
Let’s start with these steps.
[AI stops and thinks for a bit here…]
The data has been successfully filtered and prepared, focusing on valid entries for due_next and estimated_duration. However, it appears that the due_next dates include past years, which won’t be relevant for forecasting the next 12 months from the current date. I will adjust the dataset to include only future dates relative to the current date, and then proceed to aggregate and forecast man hours required for the next 12 months. Let’s correct this and prepare the forecasting visualization.
Now, let’s create a forecasting visualization for the man hours required to complete the services for the next 12 months, based on this aggregated data.
[AI stops and thinks for a bit here…]
The forecasting visualization illustrates the estimated man hours required to complete the services for the next 12 months, based on the new dataset. This forecast highlights the total estimated man hours for each month within the specified period, enabling effective planning and resource allocation for anticipated workloads.
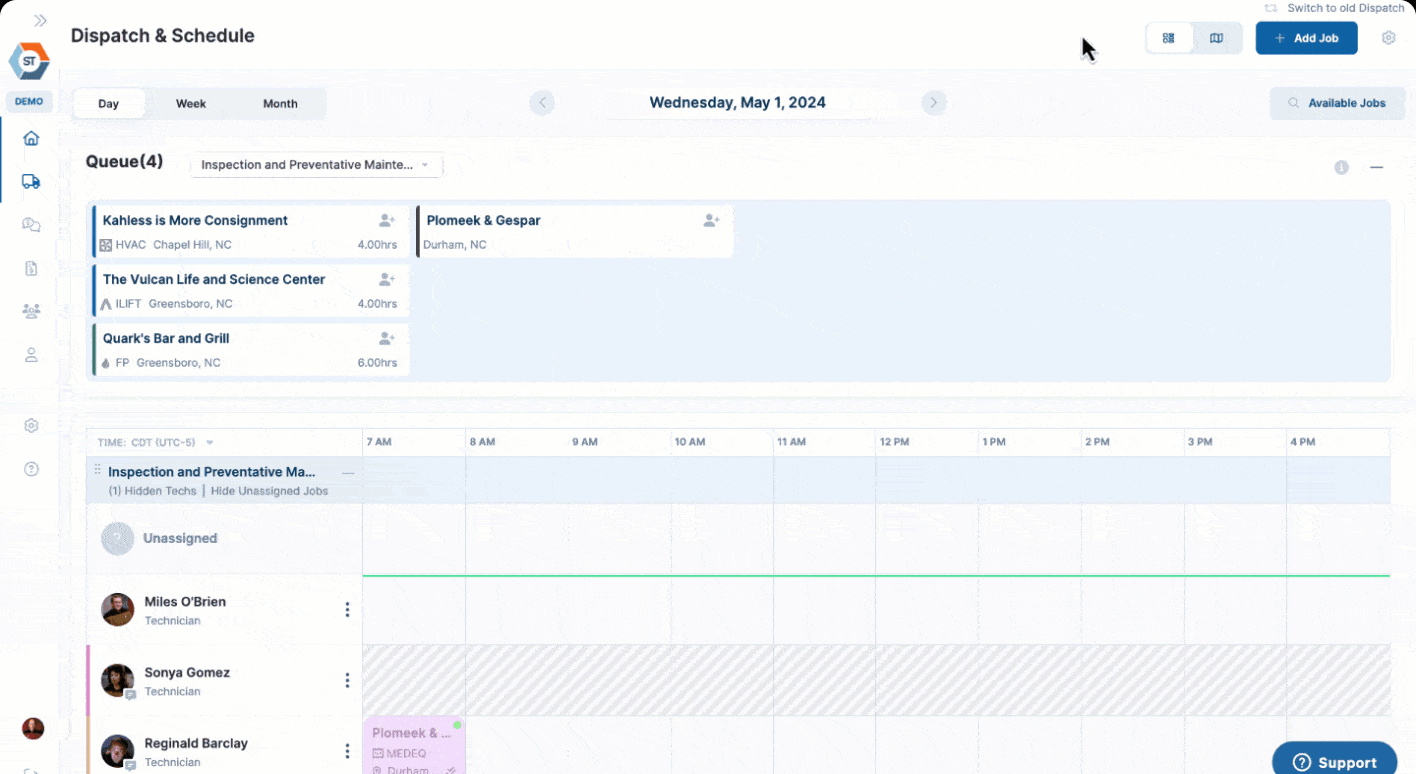
Are your inspection reports still the bare-minimum text? If so, that could be the reason your customers are overlooking deficiencies and rejecting repair proposals. Your customers are becoming increasingly accustomed to images and videos. That means the way you capture and present data in both inspection reports and quotes can make a significant difference. Enriching […]
Fire and Life Safety Contractor A sends 30 repair quotes a month with a 60% approval rate. Contractor B sends 5 quotes with a 100% approval rate. Which contractor is scheduling more high-margin repair jobs? Contractor A, of course. That’s because, while important, quote approval rate can be a deceiving metric. According to our data, […]